What is machine learning? How does it work and in which fields is it applied?
We hear more and more often about machine learning, which is now used in the most diverse fields. Let's find out what machine learning consists of and what are its fields of application.
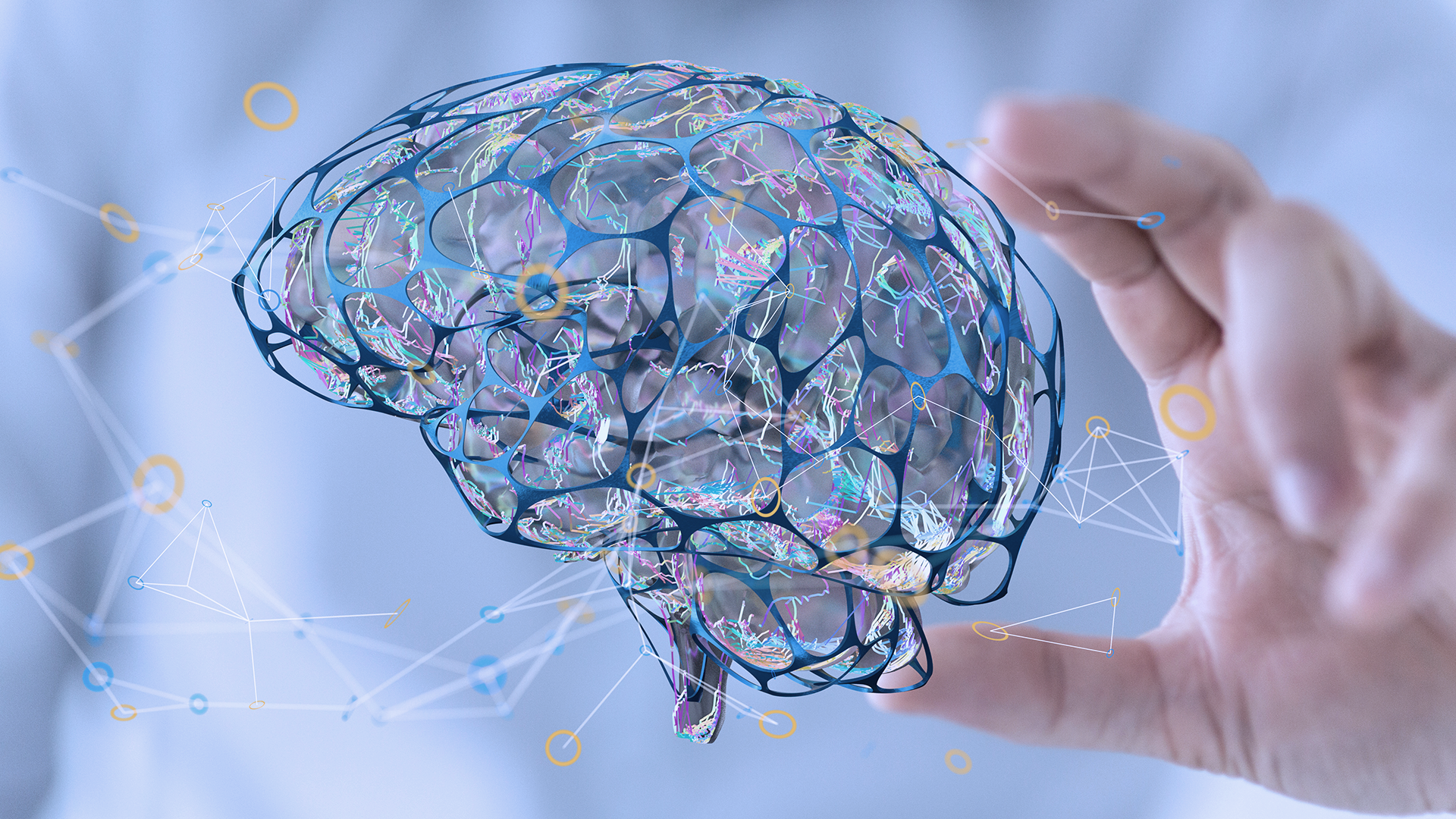
In this new appointment of the “mashfrog digital youniversity”, we tackled the issue with our specialists who explained what machine learning is and in which fields it is applied.
"Philosophical" definition of machine learning
To define machine learning, we borrowed a seemingly distant domain. In fact, taking a cue from philosophy, we can define it as the possibility of providing the machine with the ability to learn without being explicitly programmed beforehand, since it is not necessary to enter the rules each time: it will be the machine that adapts itself independently over time, learning the new rules for carrying out assigned tasks.
Difference between machine learning, artificial intelligence and statistics
First of all, it is important to differentiate machine learning from artificial intelligence, since the two terms are often confused. The latter in fact includes a much wider range of action and refers to everything that manages to emulate human behaviour in an intelligent way, respecting the rules entered while not learning anything. Machine learning is a branch of artificial intelligence that exploits notions from other sectors, such as statistics for example, allowing the machine to learn from the examples provided.
It is also important to emphasise that machine learning is more practical than statistics, because it can process and analyse much larger datasets. In fact, thanks to automatic learning and analysis of the large amount of data entered, machine learning can also be used for data mining operations, that is, the set of techniques that allows the extraction of information from large amounts of data through automatic methods.
Finally, machine learning should not be confused with the "raw" entering of data into the machine, because this offers no added value to its learning.
Difference between machine learning and traditional programming
It should be borne in mind that traditional programming is profoundly different from machine learning: in the first, the rules are written, data are entered and the machine returns responses, or outputs; on the other hand, in machine learning both the data and the expected answers are entered, but in addition the machine returns the rules it has learned.
Furthermore, from a strictly practical point of view, traditional programming requires a continuous rewriting of the rules, aimed at solving all the problems and criticalities that appear, while in machine learning the machine works independently and learns how to deal with and solve criticalities thanks to the data received.
Finally, in order to improve machine learning, it is possible to resort to so-called Reinforcement learning, which consists of entrusting the machine with rewards or penalties based on the correctness of the actions it performs.
Fields of application
Machine learning is currently applied many fields. Let's look at the most significant ones together.
Vocal and video recognition
Vocal recognition, now present on most devices, is a clear example of machine learning: after a first recording of the user's voice, the device is then able to understand the messages that are spoken, improving reception and increasingly perfecting word recognition.
Another case is that of video recording for self-driving cars. Through their sensors, the cars record what is happening around them and constantly improve the recognition of obstacles and all those elements that serve to improve their driving.
Spam filter in emails
The spam filter for email inboxes is another example of machine learning application. In this case the email client automatically recognizes what is spam from what is not. Initially, the mechanism starts from the categorisation made by users, who mark certain content and certain addresses as spam. Over time, the software absorbs more and more data, recognising the elements that constitute spam completely independently, and proceeds with automatic classification without any intervention by the human user.
In this case there is a clear difference from traditional programming where it is the user who has to rewrite the rules each time to label one or more emails as spam; with machine learning, on the other hand, the software learns the rules independently.
System of classification and generative system
Image recognition and classification is an activity in which machine learning has proven to be nothing short of fundamental. In fact, human activity would be too demanding and costly to classify a large number of images, which is why machine learning has allowed us to save time and money with an accuracy that in recent years, at least starting from 2015, has reached 96.4%, equalling the performance of a human being.
Recommendation system
Recommendation and advice systems, which are machine learning applications, have literally made the fortune of some brands. Take Netflix for example: its algorithm perfectly understands what the user's tastes are and processes them to provide relevant examples. Without this properly functioning system, the platform would be nothing more than a large archive of multimedia products.
Another case is Amazon, which collects data from searches and purchases made to provide its users with further relevant purchase advice.
AlphaGo
Finally, there is Alphago, the Google algorithm that has beaten the world champion of “Go”, the oriental board game. In this case, the computer managed by the algorithm challenged the reigning champion, independently learning the strategies to win the game, given that the programmer had only entered the rules of the game and those necessary for the learning of the machine.
Machine learning in Mashfrog: the cases of Generali Italia and AlphieWealth
The Mashfrog group has been investing in machine learning since 2016 in order to bring important innovations to different markets. As Mashfrog Group, we have collaborated with Generali Italia on the creation of Presspal, the tool that uses machine learning, artificial intelligence and sentiment analysis to create editorial content and facilitate the work of the press office. We have also applied machine learning to the field of finance with AlphieWealth, the proprietary platform that offers financial forecasts using predictive models and acquired by Ucapital24 in August 2020.
In conclusion, the functioning and cases of application of machine learning are an opportunity to be seized for different sectors of the economy of the future and we at Mashfrog are ready to take on this challenge.